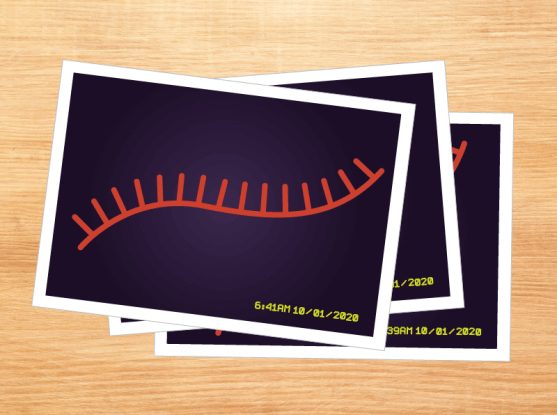
As cells grow, divide, and respond to their environment, their gene expression changes — one gene may be transcribed into more RNA at one time point, and less at another time when it’s no longer needed. In a new study, researchers at Harvard, MIT, and the Broad Institute have developed a way to determine when specific RNA molecules are produced in cells. The method, published in the journal Nature Biotechnology, allows scientists to more easily study how a cell’s gene expression fluctuates over time.
“Biology is very dynamic but most of the tools we use in biology are static; you get a fixed snapshot of what’s happening in a cell at a given moment,” said co-senior author Fei Chen, who is an HSCI Principal Faculty member, Harvard assistant professor of stem cell and regenerative biology, and core institute member at the Broad. “This will now allow us to record what’s happening over hours or days.”
To find out the level of RNA a cell is transcribing, researchers typically extract genetic material from the cell — destroying the cell in the process — and use RNA sequencing technology to determine which genes are being transcribed into RNA, and how much. Although researchers can sample cells at various times, they cannot easily measure gene expression at multiple time points.
To create a more precise timestamp, the researchers added strings of repetitive DNA bases to genes of interest in cultured human cells. These strings caused the cell to add regions of adenosine molecules — one of four building blocks of RNA — to the ends of RNA when the RNA was transcribed from these genes. The researchers also introduced an engineered version of an enzyme which slowly changed the adenosine molecules to a related molecule, inosine, at a predictable rate in the RNA. By measuring the ratio of inosines to adenosines in the timestamped section of any given RNA molecule, the researchers could elucidate when it was first produced, while keeping cells intact.
“It was pretty surprising to see how well this worked as a timestamp,” said Sam Rodriques, a co-first author of the study. “And the more molecules you look at, the better your temporal resolution.”
Improving the RNA timer
Using their method, the researchers could estimate the age of a single timestamped RNA molecule to within 2.7 hours. But when they looked simultaneously at four RNA molecules, they could estimate the age of the molecules to within 1.5 hours. Looking at 200 molecules at once allowed the scientists to correctly sort RNA molecules into groups based on their age, ordering them along a timeline with 86% accuracy.
“Extremely interesting biology, such as immune responses and development, occurs over a timescale of hours,” said co-first author Linlin Chen. “Now we have the opportunity to better probe what’s happening on this timescale.”
The researchers found that the approach, with some small tweaks, worked well on various cell types: neurons, fibroblasts and embryonic kidney cells. In one application, they are planning to now apply the method to study how levels of gene activity related to learning and memory change in the hours after a neuron fires.
The current system allows researchers to record changes in gene expression over half a day. The team is now expanding the time range over which they can record gene activity, making the method more precise, and adding the ability to track several different genes at a time.
“Gene expression is constantly changing in response to the environment,” said co-senior author Edward Boyden of MIT. “Tools like this will help us eavesdrop on how cells evolve over time, and help us pinpoint new targets for treating diseases.”
This story was originally published on the Broad Institute website on October 19, 2020.
Source article: Rodriques, S. G., Chen, L. M., et al. (2020). RNA timestamps identify the age of single molecules in RNA sequencing. Nature Biotechnology. DOI: 10.1038/s41587-020-0704-z
This research was supported by the National Institutes of Health, the Paul G. Allen Frontiers Group, the Burroughs Wellcome Fund, the Schmidt Fellows Program at the Broad Institute, John Doerr, the Open Philanthropy Project, the HHMI-Simons Faculty Scholars Program, the U.S. Army Research Laboratory and the U.S. Army Research Office, the MIT Media Lab, Lisa Yang, the Hertz Graduate Fellowship, and the National Science Foundation Graduate Research Fellowship Program.